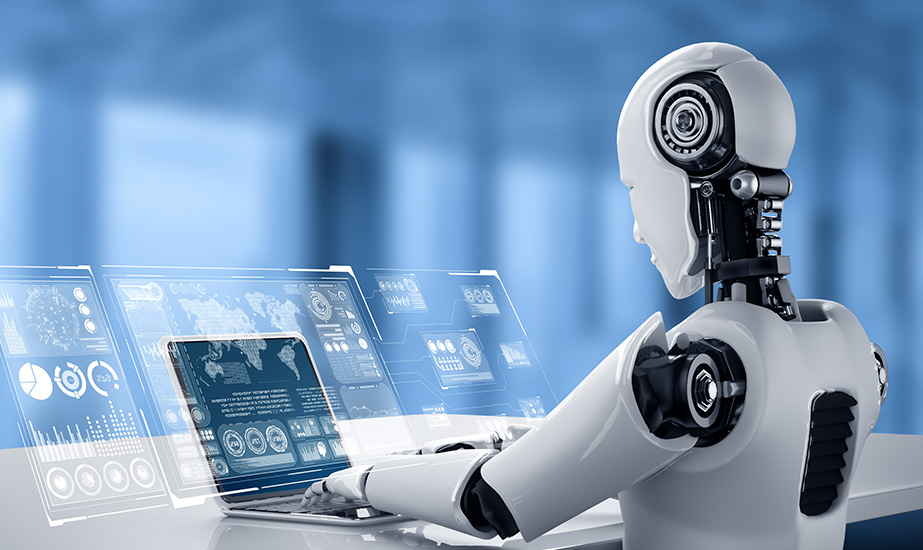
At Bizprules Inc, our AI workflow is designed to seamlessly integrate cutting-edge technology with business processes to drive innovation and efficiency. We start by identifying key business challenges and opportunities where AI can deliver the most value. Our expert team then collects and preprocesses relevant data, ensuring it is clean and ready for analysis.
Using advanced machine learning algorithms and data analytics, we develop tailored AI models that are rigorously tested and validated. These models are then deployed into production environments, where they continuously learn and adapt to new data, providing actionable insights in real time.
Our AI solutions are fully scalable and customizable, allowing us to meet the unique needs of each client. With ongoing monitoring and optimization, Bizprules Inc ensures that our AI-driven strategies consistently deliver superior performance and drive sustainable growth for your business.
AI Workflow: A Comprehensive Guide
1. Problem Definition
- Objective: Clearly define the problem you want to solve with AI.
- Scope: Identify the scope and constraints of the project.
- Stakeholders: Engage with stakeholders to understand their requirements and expectations.
2. Data Collection
- Data Sources: Identify and gather data from relevant sources.
- Data Types: Ensure a variety of data types (structured, unstructured, images, etc.).
- Volume and Quality: Ensure you have enough data of good quality to train the AI model.
3. Data Preparation
- Cleaning: Remove duplicates, handle missing values, and correct errors.
- Transformation: Convert data into a suitable format for analysis.
- Normalization: Scale the data to ensure uniformity.
- Splitting: Divide data into training, validation, and test sets.
4. Exploratory Data Analysis (EDA)
- Descriptive Statistics: Summarize the main features of the data.
- Visualization: Use graphs and charts to identify patterns and insights.
- Correlation Analysis: Determine relationships between different variables.
5. Feature Engineering
- Feature Selection: Identify the most relevant features for the model.
- Feature Creation: Generate new features from existing data.
- Dimensionality Reduction: Reduce the number of features while maintaining important information.
6. Model Selection
- Algorithm Choice: Select the appropriate machine learning algorithms (e.g., regression, classification, clustering).
- Baseline Model: Start with a simple model to set a performance benchmark.
- Complex Models: Experiment with more